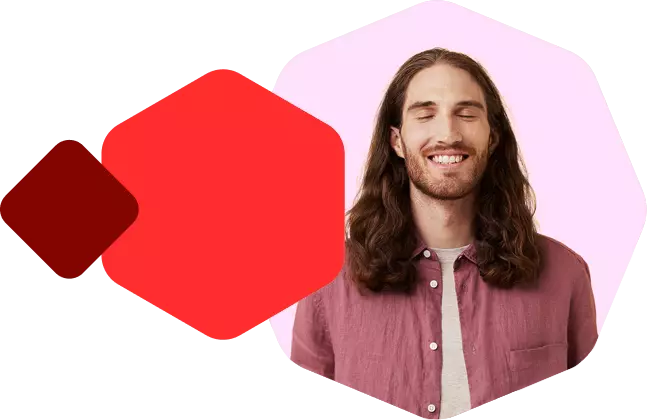
The website is currently not available
The website you want to visit is currently not available. This may be due to that the website is being developed, that maintenance is underway or that the website is closed due to some other reason.
Domain owner, please see your contact address (email) or contact support@loopia.com for more information.
Webbplatsen går ej att nå för tillfället
Webbplatsen du vill besöka är för tillfället inte tillgänglig. Detta kan bero på att sidan är under utveckling, att underhåll görs eller att sidan av annan anledning är stängd.
Domänägare, se din kontaktadress (e-post) eller kontakta support@loopia.se för mer information.
Nettsiden kan ikke nås for øyeblikket
Nettsiden du vil besøke er for øyeblikket ikke tilgjengelig. Dette kan komme av at siden er under utvikling, vedlikehold utføres eller at siden av en anledning er stengt.
Domeneier, se din kontaktadresse (e-post) eller kontakt support@loopia.no for mer informasjon.